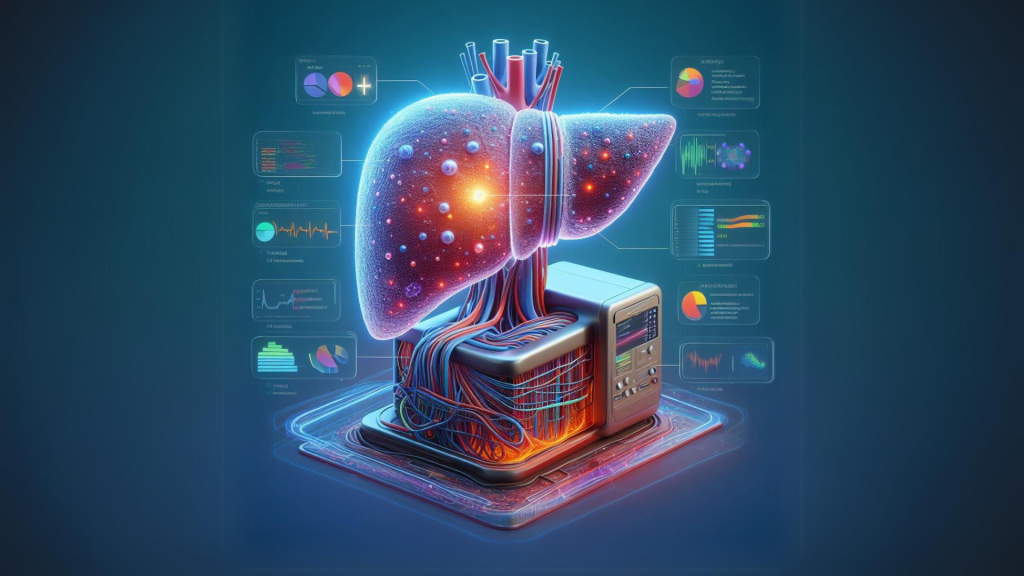
The intersection of machine learning (ML) and healthcare continues to revolutionize medical practices, with recent advancements focusing on predicting cancer risk in patients with metabolic dysfunction-associated steatotic liver disease (MASLD). Researchers from UC Davis Health have developed an ML tool to identify individuals at heightened risk of developing hepatocellular carcinoma (HCC), a prevalent form of liver cancer, within this patient population.
Understanding the ML Model:
The ML model, built by the UC Davis research team, harnesses predictive analytics to assess the risk of HCC development in MASLD patients. By analyzing clinical variables, the model effectively predicts which patients are more likely to face the risk of liver cancer, enabling proactive monitoring and early intervention strategies.
Validation and Performance:
The researchers evaluated nine initial ML algorithms, ultimately shortlisting five based on superior performance metrics such as accuracy, specificity, and sensitivity. Through rigorous validation using data from UC San Francisco Medical Center, the Gradient Boosted Trees algorithm emerged as the top performer, showcasing exceptional predictive capabilities.
Key Predictors and Insights:
The analysis identified several key risk factors associated with HCC development, including advanced liver fibrosis, cirrhosis, hypertension, high cholesterol, and abnormal levels of bilirubin and alkaline phosphatase (ALP). Notably, the model highlighted patients with low Fibrosis-4 Index (FIB-4) but elevated cholesterol, hypertension, and bilirubin levels as being at high risk for HCC, challenging conventional risk assessment practices.
Integration and Future Directions:
The research team envisions integrating the ML model into electronic health records (EHRs) to aid clinicians in identifying MASLD patients at increased risk for HCC. Future enhancements may involve incorporating additional data, such as clinical notes, through natural language processing (NLP), to further refine the predictive accuracy of the model.
Implications and Considerations:
While ML holds promise in enhancing risk prediction for various medical conditions, including cancer, robust validation and continuous refinement are paramount. The findings underscore the importance of clinical validation to ensure the reliability and effectiveness of predictive analytics tools in real-world healthcare settings.
The application of ML in predicting HCC risk among MASLD patients represents a significant advancement in precision medicine. By leveraging predictive analytics, clinicians can proactively identify individuals at heightened risk for liver cancer, facilitating timely interventions and improving patient outcomes. As ML continues to evolve, its integration into clinical practice holds immense potential for enhancing risk stratification and personalized patient care.